Conformal Cone Parameterization Through Optimal Control
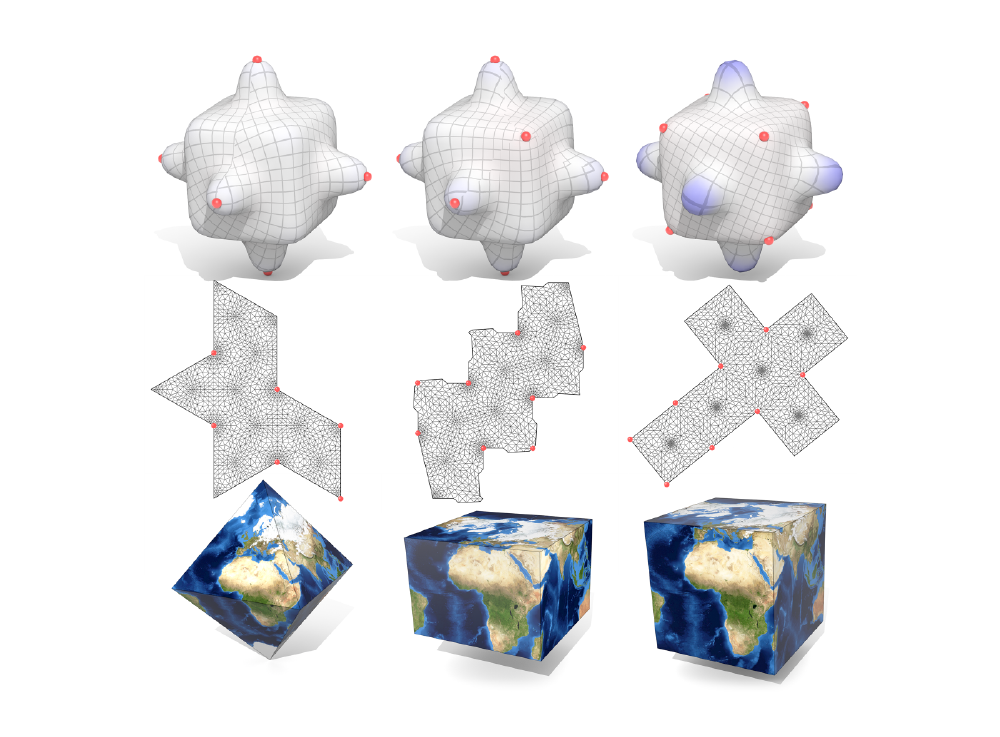
We cast the problem of finding optimal cone singularities as an optimal control problem. It is still a challenging open problem to find the optimal configuration of quantized cone singularities, even if the positions of the cone singularities are fixed.
Surface parameterizations with low metric distortion are essential for a wide variety of
applications ranging from geometry processing, to digital manufacturing, to machine
learning. Conformal param- eterizations are easy to compute and exactly preserve angles,
but can significantly distort areas. Cone singularities have been introduced as a way of
mitigating area distoriton, but finding the configuration of cones that best reduces
distortion is notoriously difficult. This thesis develops a simple strategy that
provides globally optimal configurations of singularities, in the sense that the
configuration minimizes the total area distortion among all possible conformal cone
configurations (number, placement, angle) that have no more than a fixed total cone
angle. In practice, our optimal cone configurations can yield dramatically lower area
distortion than those found via existing heuristics. Moreover, the approach can be
extended to allow user-defined notions of importance, find the best flattening with a
convex or polygonal boundary, and produce solutions with only positive cone angles.
Our approach can be summarized as follows: the cone singularity placement problem is relaxed to a convex optimization problem over the space of finite signed Radon measures with a sparsity inducing regularization. By utilizing Fenchel-Rockafellar duality we obtain an equivalent formulation over some usual function spaces, which are easily and properly discretized using finite elements. Computing the optimal configuration of cones amounts to solving a sequence of sparse linear systems easily built from the usual cotangent Laplacian. The experimental results presented in this thesis provide some evidence that simple sparsity inducing norms may be widely applicable to problems arising in computer graphics and geometry processing.
Our approach can be summarized as follows: the cone singularity placement problem is relaxed to a convex optimization problem over the space of finite signed Radon measures with a sparsity inducing regularization. By utilizing Fenchel-Rockafellar duality we obtain an equivalent formulation over some usual function spaces, which are easily and properly discretized using finite elements. Computing the optimal configuration of cones amounts to solving a sequence of sparse linear systems easily built from the usual cotangent Laplacian. The experimental results presented in this thesis provide some evidence that simple sparsity inducing norms may be widely applicable to problems arising in computer graphics and geometry processing.